Abstract
Objects in the world are typically defined by contours and local features separated by extended featureless regions. Sparsely
sampled profiles were therefore used to evaluate the cues involved in localizing objects defined by such separated features (as opposed
to typical Vernier acuity or other line-based localization tasks). Objects, in the form of Gaussian blobs, were defined at the
sample positions by luminance cues, binocular disparity cues or both together. Remarkably, the luminance information in the
sampled profiles was unable to support localization for objects requiring interpolation when the perceived depth from the luminance
cue was cancelled by a disparity cue. Disparity cues, on the other hand, improved localization substantially over that for luminance
cues alone. These data indicate that it is only through the interpolated depth representation that the position of the sampled object
can be recognized. The dominance of a depth representation in the performance of such tasks shows that the depth information is
not just an overlay to the 2D sketch of the positional information, but a core process that must be completed before the position of
the object can be recognized.
Journal
Volume
Number of Pages
Year of Publication
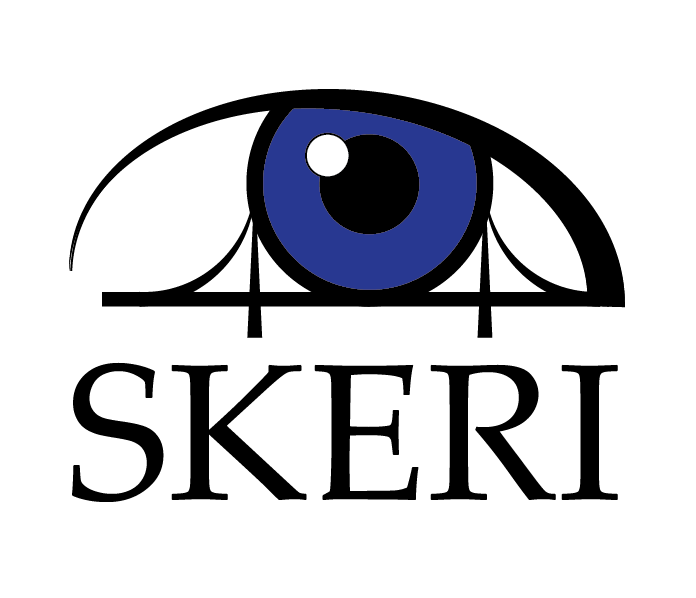